Gen AI adoption journey: what matters at each stage and how to succeed
5 steps on a typical journey, and what matters varies step by step and distilled patterns common to the most successful of them
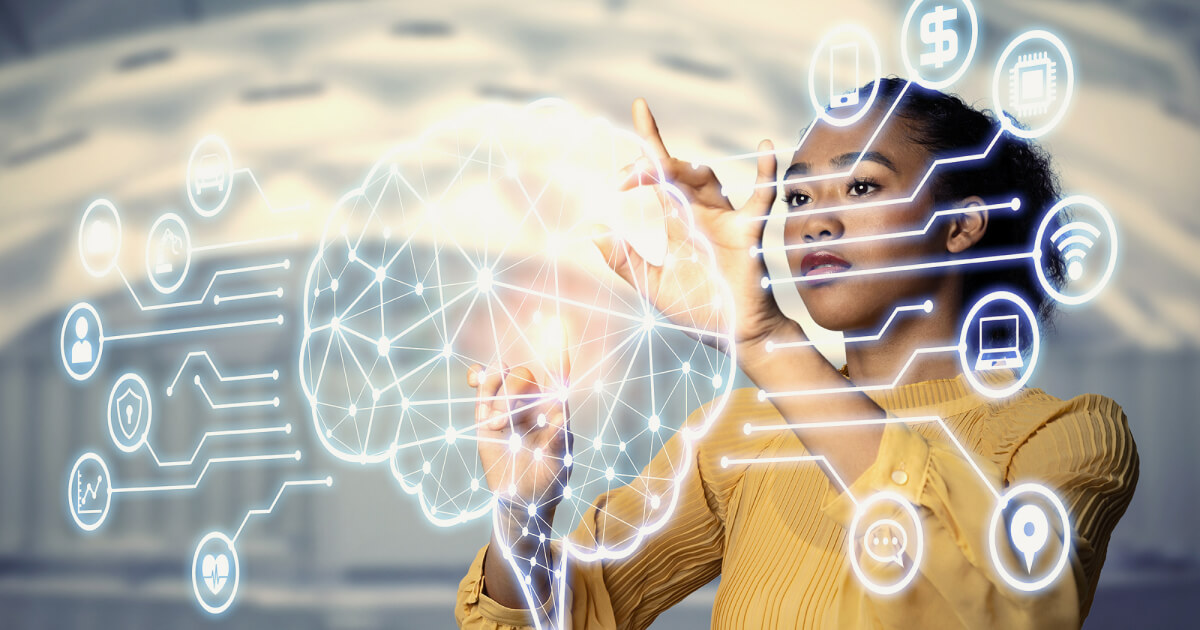
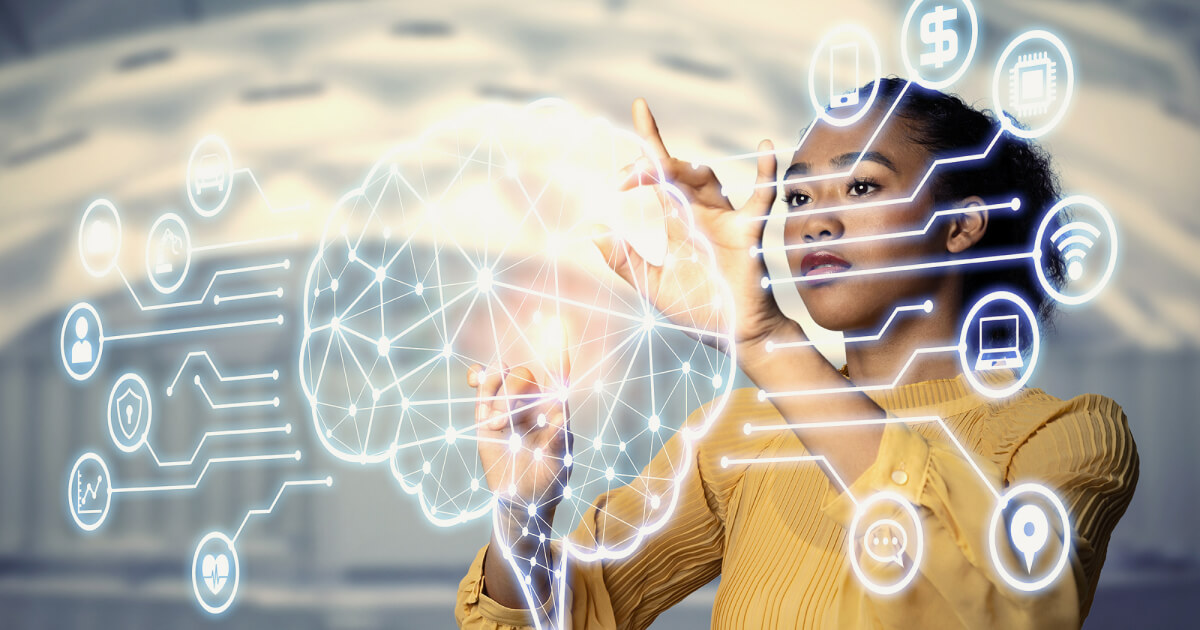

Adopting Gen AI apps is a journey where you don’t know the destination when you embark on it. The tech is evolving so fast and the use cases are still limited largely by our own imagination. NROC Security interviewed tens and tens of organizations to learn how people driving Gen AI adoption think about the road they are on.
From those discussions, we distinguished 5 steps on a typical journey, and what matters varies step by step and distilled patterns common to the most successful of them.
Exploration. Top down and bottom up ideation of use cases and trials of interesting technologies. Small number of very active users and apps being tried and quickly discarded. What matters: Allow some exploration with the company computers. If all is blocked, people either experiment with private laptops and you lack visibility from day one. If people are kept from learning, that can put your company behind the competition right out of the gate.
Evaluation. Three things are happening here. The first apps (most often ChatGPT) start to be more widely used in the organization. The AI working group gets systematic in evaluating use cases with the risks and cost benefits. Finally, policies are written and end user training organized. What matters: Visibility to what is actually done with Gen AI is key at this stage. It reduces the pressure to try to mitigate Gen AI risks contractually with the vendor (which is anyway hard with the new tech and unknown vendors) and it allows identification of those use cases that have widespread potential. Equally important is to internally spread the word about those users that are already realizing business value from Gen AI.
Pilots to rollouts. The first pilots look to be of value and the visibility is showing usage patterns that are in line with the acceptable use policy. Time to roll out the first use cases to broader user groups. While the pilot group was probably vetted and well trained, now the user group starts to resemble the average business users. The use is still very restricted. Typically no proprietary data is to be used with Gen AI. What matters: Basic policy enforcement with auditable tracking is usually a prerequisite for a broader rollout. At this point, there often is a need to prove (or at least duty to know) that the policies are being followed. An investigation process via Security Operations is relevant at this stage, so that investigation into an individual's prompts of Gen AI can be handled using a proper process.
Integration with proprietary data and workflows. There’s a Finnish proverb that roughly translates to ‘hunger grows while eating’. Same here. The public-only policy feels restrictive and the information copy/pasting manually feels … manual. The next step is to seek more value from Gen AI and put company data to work. This may mean purchasing ‘dedicated instances’, ‘isolated models’ or ‘enterprise versions’, or then just vetting the app vendor a notch more and lifting some restrictions on allowed data. At this stage, the API connections are implemented to ground the Gen AI prompts with uploaded or crawled material, and Gen AI outputs are directly integrated into the business process. What matters: Use cases tend to drift when the technology develops and organizational ambition increases. Monitoring for data flows and integrations becomes important. At this stage, a business user in a downstream process may receive information that was created by Gen AI and needs to be made aware of that. Ability to trace back and review how a particular end product was made is a cornerstone of responsible use of AI.
Offboarding discontinued apps. Every effective exploration and piloting results in many discarded ideas and apps. A more effective app emerged, or a business use case was not valuable enough to warrant continuation. What matters: Company data is likely left behind in all of the discarded apps. While contractual data removal obligations may be effective, it is still important to have a record of any usage of a particular app, up to the level of the interactions. If the app is breached down the road, this information allows your organization to size the exposure.
The journey looks different for every organization. For example, heavily regulated companies are less ready to explore, whereas somebody else may have difficulties distinguishing a promising pilot from all ongoing trials.
What then separates the more successful early adopters from the rest? Two differences in mindset:
- They do not assume upfront they know the destination. They encourage and facilitate organizational learning, see with curiosity what use cases and apps get traction, and amplify those to the rest of the organization
- They think ahead a step or two and build capabilities already while the organization is still scouting for the best use cases. They believe that there’s something valuable in Gen AI, and if they don’t get ready to implement, their competition will.
Somebody compared Gen AI to the industrial revolution, the other to the invention of farming. Wherever you are on the journey, it is bound to be a transformational one.
NROC was founded to enable business users to safely take advantage of Generative AI technologies. We all are in the early innings of this, and look forward to innovating with our customers. More information about NROC Security please see our website at www.nrocsecurity.com